ABOUT
Conifold Theory allows us to understand consciousness in terms of physical processes taking place in the brain. The better we understand how consciousness arises naturally, as information is encoded by neurons across the cerebral cortex, the better we can understand how we, as individuals, can maintain healthy cognition and behavior.
A scientific understanding of consciousness
​
By understanding the physical basis of consciousness, we can better relate neural activity to mental processes. And by understanding the relationship between the brain and the mind, we are better equipped to treat the unique challenges of mental health practice. When we have a clear picture of how conscious information processing occurs, we can improve the effectiveness of information processing. The goal of putting this theory into practice is to help individuals better engage with consciousness - to perceive the world, understand the world, and act with purpose.
Building a theoretical framework should aid mental health practitioners in three specific ways. Firstly, having a good understanding of how the brain works may help to identify perceptual blind spots and improve situational awareness. Secondly, an appreciation of neural information processing may prompt individuals to more consciously deliberate over incoming data, leading to more complete and accurate mental models of the world. And thirdly, an awareness of how the brain drives action may guide individuals to choose behaviors that better achieve goal-directed outcomes. Applying neuroscience in mental health practice should therefore help people to better navigate their reality.
​
An Introduction to Conifold Theory
​
There is no evidence of perceptual experience or volitional behavior in the absence of cortical neuron activity. An understanding of consciousness therefore requires some explanation of how thought, perception, memory and beliefs are related to neural signaling in the brain. This is exactly what Conifold Theory aims to accomplish.
​
In studying the physical relationship between the brain and the mind, researchers have found that essentially the brain is an information-encoding structure. Researchers at the Western Institute for Advanced Study have taken this concept a step further, demonstrating that mental states are the information content that is encoded by the neural network. Because energy must physically be expended to create information [1, 2], the laws of thermodynamics can be applied to quantify the amount of energy used to generate information in each computational cycle and the amount of work done to store information in the brain [3, 4]. The mechanics underpinning that encoding process are described in terms of ion flux across the neuronal membrane [5]. Furthermore, information that is physically encoded on a neural network structure generates a holographic projection of information content [6]. This theoretical framework provides greater explanatory power than previous theories [7] and yields dozens of specific and falsifiable predictions which can be investigated by formal scientific experimentation [8]. The papers cited here form the foundation of the theory.
Nature or Nurture?
​
We are products of both nature and nurture. While the general structure of our brains is developmentally programmed, that wiring is constantly remodeled over the course of our lifetimes. As we encode our experiences into the very structure of our brains, we thermodynamically favor patterns of activity which have occurred previously [9, 10].
The remodeling of neural connections is a structural change, but this process has a discrete effect on how we function. After we have encoded an experience in the brain, our neural pathways are set up to expect that series of events to happen again. As a result, we are both products of our biology and our environment. Our amazing genes both build the basic structure of the brain and permit this basic structure to be remodeled. However, we do require experiences to develop an understanding our world. Experience provides us with information about the world to encode!

The neural basis of perceptions, memories, and decisions​
​
Simply put, conscious perceptual experience is not possible without neural activity. That said, perceptual experience is something categorically different from neural activity. The physical nature of this correspondence is described in Conifold Theory, with the brain as an encoding structure and the percept as information content. This natural phenomenon allows us to experience a cohesive experience of the world, so that we may act effectively within the world.
Thousands of studies have demonstrated how neural activity is tied to perceptual experience. The experience of seeing colors and shapes is tied to neural activity in visual cortex [11, 12]. The experience of hearing is tied to neural activity in the auditory cortex [13, 14]. Not only are characteristic patterns of neural activity paired with the timing of perceptual experience, but different patterns of neural activity are correlated with different experiences [15, 16].
Memories are also tied to neural activity. As we learn associations between a context and an event, or an important event that tends to follow a more innocuous event, the patterns of neural activity which encode that information become strengthened. Synaptic connections that encode reliable information will be reinforced, while synaptic connections that are not as useful may degrade. The nervous system is constantly remodeling itself, as we learn about the world [9, 10]. These structural modifications favor the same patterns of activity to repeat – for example, a similar combination of electrical signals will fire throughout the brain when a person enters a familiar context. In this way, the brain physically encodes memories in its very structure.
And thirdly, decision-making is tied to neural activity. In higher regions of cortex, multiple sensory modalities combine to influence motor output [17, 18]. When neurons reach the threshold for sending a signal, this event corresponds to the experience of making a decision [19, 20]. The behavior that results is an output of that neural coding.
In summary, perception, memories, and decisions are encoded in neural activity. And to a great extent, neuroscience has uncovered correspondences between these cognitive phenomena and characteristic changes in neural activity. However, neural activity is not the same as perception, memory, and decision-making. There is currently an explanatory gap, and this is where Conifold Theory proves useful (Figure 1). This scientific framework aims to explain what consciousness is, and how it is related to neural activity.

Neurocognition as a physical, computational process​
​
The field of neuroscience has provided great insight over the past 200 years on how different brain regions contribute to the phenomena of perception, memory, and executive control of behavior. However, neuroscience has been unable to explain how neural activity gives rise to the qualitative, experiential nature of mental phenomena – until recently.
Conifold Theory is a neuroscience-based framework for understanding this relationship. In Conifold Theory, the brain is an encoding structure and qualitative perception is the information content, integrated across the entire neural network and holographically projected into a higher-dimensional space. This information content is only accessible to the system that encodes the information, and it is continuously updated in real time as the neural activity changes from moment to moment. Over a lifetime, the integration of all these individual neural network states - each correlated with qualitative content - forms a cumulative information set. That accumulated information is the set of beliefs about the world and the role of the self within that world, which have been collected through lived experience.
Information has a mathematical definition. It is the sum of all possible system states which form over some time period [21, 22]. Conifold Theory uses the term in this strict mathematical sense. This may seem to contradict the common use of the term, in which information is the meaning gleaned from a dataset. This definition of information is also applied in Conifold Theory. The core principle of the theory is that individuals produce information in the mathematical sense, by physically generating disorder or entropy (physically corresponding to a set of possible neural network states). Within a computational cycle, we then parse this information content for correlations or consistencies. In doing so, we reduce the amount of disorder in the system to create order or meaning from this physical dataset. This process corresponds to identifying the optimal system state, or the best way to encode the incoming information within the neural network. In some cases, we may remain uncertain of what is true about the world, while in other cases, we may be able to reduce uncertainty by choosing the most likely scenario to be 'true'. This process of parsing messy information and deciding on the most likely true state of the world is a computational process – we are both selecting the information content with the most meaning and we are selecting the system state that encodes the information content with the most meaning. And so, conscious information processing involves both generating information (in the mathematical sense) and compressing that information (to glean meaning from this dataset).
Consciousness, in this neuroscientific framework, is a cyclical process of information generation and reduction which is tied to neural activity. This is not to say that ONLY humans can be conscious – but there are specific structural and functional requirements for consciousness, which plants and rocks and laptop computers do not meet. From the perspective of Conifold Theory, plants and rocks and other things without a complex probabilistic neural network simply cannot experience perception or choose how to behave. However, other animals may meet these requirements, and eventually, engineered neural networks may meet these requirements as well.
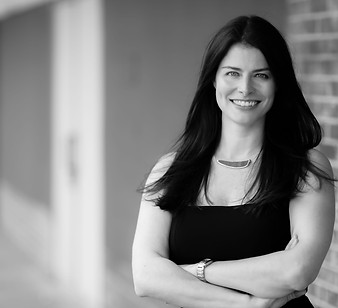
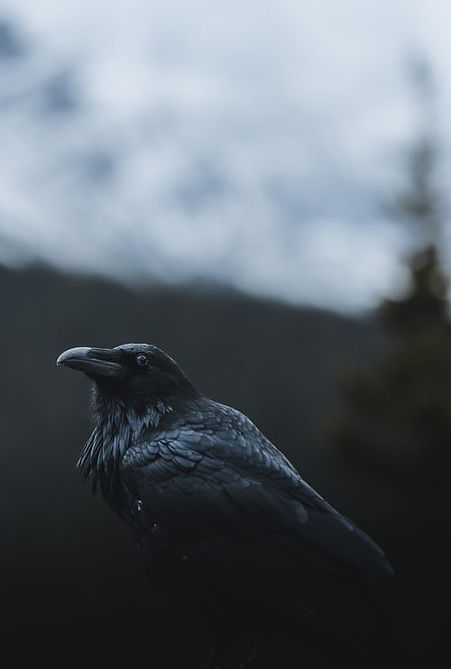
An overview of thermodynamic information processing​
Some quantity of energy must be physically expended to create information [1]. In accordance with Landauer’s principle, this energy is not lost, but rather is conserved as it is converted into entropy. While the information is encoded within the neural network, in the pattern of electrical signals pulsing through the neural network structure, the actual information content is projected into a higher dimensional space.
During each computational cycle, new probable system states emerge and information entropy is physically generated along the time axis. Within a single computational cycle, the constraints on the system are calculated, and a thermodynamically-favored state is identified. The completion of the cycle is achieved with a compression of information entropy, as the most likely system state is resolved and actualized. In that instant, all non-actualized states are reduced, and the recovered energy is released back into the system.
Consciousness can therefore be described in terms of thermodynamics, with energy being exchanged to create information entropy and do work in the system; in terms of quantum mechanics, with the probabilistic behavior of particles cyclically generating and compressing information; and in terms of holography, with the information that is encoded on the neural membrane structure being projected into a cohesive stream of perceptual experience. In each description, the information encoded by a neural network is limited by the amount of energy available to the system.
A rival energetic cost function leads us to explore our environment and exploit the knowledge we have gained​
​
We collect data about our surrounding environment through the senses, and encode these data in our brains. That process of probabilistic coding physically generates information. This information is encoded by neurons in the brain, which integrate random electronic noise with upstream signals. Any correlations or consistencies within this dataset - or with previously-collected data - naturally compresses that quantity of information. So, as we interact with our surrounding environment, our brains predict the most likely ‘true’ state of the local environment and encode this prediction. This act of perception may be accurate or inaccurate - if new information conflicts with previously-collected information, the new information may not be accepted, and an inaccurate perception of reality will result.
In this framework, consciousness is an act of computation. Neurons in the central nervous system are acting as qubits, which calculate the probability of reaching a state change, rather than classical computing units, which exist always in a simple on-or-off state. In this way, neurons are able to use quantum-level noise to calculate the most likely system state overall. Within a single computational cycle, the neural network will resolve into the most thermodynamically favored state. This computational process is paired with a semantic statement about the external environment. This predictive model about the current state of the environment is then validated by orienting toward a stimulus, to collect more information through the senses. These semantic truth statements are held in working memory, so they can be integrated with subsequent neural network states to compute any syntactical relationships between events. A repeated pairing of action and consequence will solidify a predictive model of cause and effect, allowing the individual to build a cognitive model of how the world works. These predictive cause-effect models of the world can later be used for reference in subsequent situations, as the individual attempts to predict likely outcomes based on present incoming sensory information and past experiences of similar events.
Since unlikely or unfamiliar system states may hold predictive value, it may be favorable for the system to expand the quantity of information entropy and accommodate a new system state, even at an energetic cost, as this may reduce uncertainty for the organism in the longer term. Any information held in memory will be parsed for predictive value, and any sequences of neural network states, encoding cause-effect relationships about reality, will be stored in memory. Interestingly, the more predictive value in the information set, the more meaning can be extracted. This leads to more information compression and more free energy being released to do work. That work involves triggering spontaneous behavior and spontaneous remodeling of the neural network to encode memory. As the system works to remodel itself into a more organized state - achieving more effective behavior in a given situation - the disorder of the system is reduced, less uncertainty or entropy is produced in that context, and more free energy is available to the system. That is why it is so easy to navigate familiar situations, and so tiring to navigate new or uncertain situations.
We ourselves, as thermodynamic computing systems, will reduce information entropy, by favoring previous patterns of neural activity. Hewing to familiar situations maximizes efficiency and makes use of previously-gained predictive value. We will also expand information entropy, by engaging in exploratory behavior and storing any new, useful information into memory. Expending energy to explore new ideas or new situations provides the opportunity to gain predictive value or additional knowledge about the world. So we operate on a rival cost function - saving energy by staying in familiar situations and expending energy to learn more about our world. These two computational processes perform in opposition - the generation of entropy is thermodynamically unfavored, but it is favored if the new information offers predictive value. That is because, whenever a true statement about the world is realized, all other possible arrangements fall away, information is compressed, understanding is gained, and this reduction of energy within a computational cycle releases free energy into the system. That energy is then used to remodel the system state, to thermodynamically favor that pattern of neural activity to occur again. Now the situation is familiar and can be easily navigated in the future. And so, during learning, a neural network will self-organize into a highly-ordered state, as it computes the predictive value or potential meaning of incoming data.
Neurological limits to perception, and the necessity of seeking truth to navigate the world effectively
​
Just because we cannot access reality directly does not mean there is no objective reality. It just means that we have imperfect ways of perceiving it. Only a narrow range of the electromagnetic spectrum can be sensed by our eyes, and only a narrow range of mechanical waves can be sensed by our ears. There may be entire types of events in the universe that we have no knowledge of, because we have not evolved senses to collect these data. But just because our knowledge of reality is necessarily incomplete does not mean there is no reality or that we cannot observe it. By contrast, we are equipped to learn about our world, and doing so helps us to better navigate within our world.
By applying Conifold Theory in practice, we can consciously expand our perception to include data we may have previously ignored. We may also choose to expand the number of mental models available to us, existing in a productive period of uncertainty which permits us to deliberate between several paths forward. And finally, we may consciously select a behavior that is predicted to have the best outcome, in terms of achieving our goals. By tackling each stage of information processing step by step, we can engage in conscious perception, conscious mental modeling of our world, conscious decision-making, and conscious implementation of behavior.
Clinical applications of this theoretical framework
​
This new theoretical framework provides a cohesive framework for understanding the relationship between the brain and the mind - a critical goal in psychiatry. It turns out to be enormously useful to map this computational process. By identifying the critical faults that occur each step in the process, we can identify the symptoms that emerge from this dysfunction. Each of these critical faults correspond with a common neuropsychiatric condition (Table 1).

Conclusions​
​
Conifold Theory is a framework for explaining consciousness in terms of physical information processing. Within this theory, the brain is an information-encoding structure, while perception and beliefs are the information content encoded by that structure. Because energy must be expended to create information, we must physically devote energy to perceive reality and to construct an understanding of reality.
In this view, our memories and beliefs are the information we have accumulated over a lifetime, and because they are useful to us, we do not easily dismantle them. Whenever redundant information is identified, no additional energy must be expended to accommodate it, and so these data are easily accepted. Whenever conflicting information is confronted, additional energy must be expended to accommodate it, so it is often easier to discard these data as anomalous or incorrect. If ignoring information or sticking to old pathways presents challenges for the individual to effectively navigate their reality, then it becomes necessary to spend additional time and energy engaging conscious perception, forming new mental models of the world, articulating goals, and choosing behaviors which have more positive outcomes.
In accommodating the physical and energetic requirements of conscious information processing, the principles of Conifold Theory can be usefully employed in mental health practice to improve both individual well-being and behavioral outcomes.

References Cited​
1. R. Landauer, Irreversibility and heat generation in the computing process. IBM Journal of Research and Development, 1961. 5: p. 183-91.
2. A. Berut, A. Arakelyan, A. Petrosyan, S. Ciliberto, R. Dillenschneider, E. Lutz, Experimental verification of Landauer's principle linking information and thermodynamics. Nature, 2012. 483(7388): p. 187-9.
3. S. Still, D.A. Sivak, A.J. Bell, G.E. Crooks, Thermodynamics of prediction. Physical Review Letters, 2012. 109(12): p. 120604.
4. E.A. Stoll, Random electrical noise drives non-deterministic computation in cortical neural networks. doi:10.1101/2022.12.03.518981.
5. E.A. Stoll, The mechanics underpinning non-deterministic computation in cortical neural networks. doi:10.1101/2022.12.03.518983.
6. E.A. Stoll, Modeling electron interference at the neuronal membrane yields a holographic projection of representative information content. doi:10.1101/2022.12.03.518989.
7. E.A. Stoll, The explanatory power of Conifold Theory. Article in preparation.
​
8. E.A. Stoll, The neuroscientific predictions of Conifold Theory. Article in preparation.
​
9. D.O. Hebb, The Organization of Behavior. New York: Wiley & Sons, 1949.
​
10. D.H. Edwards, S.R. Yeh, F.B. Krasne, Neuronal coincidence detection by voltage-sensitive electrical synapses. Proc Nat Acad Sci, 1998. 95(12): p. 7145-50.
​
11. S.G. Solomon and P. Lennie, The machinery of colour vision. Nat Rev Neurosci, 2007. 8(4): p. 276-86.
​
12. H. Zhou, M. Davidson, et. al., Spatiotemporal dynamics of brightness coding in human visual cortex revealed by the temporal context effect. NeuroImage, 2020. 205: p. 116277.
​
13. N.R. Swerdlow, T.D. Blumenthal, A.N. Sutherland, E. Weber, J.A. Talledo, Effects of prepulse intensity, duration, and bandwidth on perceived intensity of startling acoustic stimuli. Biol Psychol, 2007. 74(3): p. 389-95.
​
14. J.D. Chambers, D. Elgueda, J.B. Fritz, S.A. Shamma, A.N. Burkitt, D.B. Grayden, Computational Neural Modeling of Auditory Cortical Receptive Fields. Front Comput Neurosci, 2019. 13: p. 28.
​
15. D. Pins and D. Ffytche, The neural correlates of conscious vision. Cereb Cortex, 2003. 13(5): p. 461-74.
​
16. J.S. Snyder, C.M. Schwiedrzik, A.D. Vitela, L. Melloni, How previous experience shapes perception in different sensory modalities. Front Hum Neurosci, 2015. 9: p. 594.
​
17. J.M. Beck, W.J. Ma, et. al., Probabilistic population codes for Bayesian decision making. Neuron, 2008. 60(6): p. 1142-52.
​
18. P. Mostert, P. Kok, F. P. de Lange, Dissociating sensory from decision processes in human perceptual decision making. Sci Rep, 2015. 5: p. 18253.
​
19. T.D. Hanks, M.E. Mazurek, R. Kiani, E. Hopp, M.N. Shadlen, Elapsed decision time affects the weighting of prior probability in a perceptual decision task. J Neurosci, 2011. 31(7): p. 6339-52.
20. P. Kok, G.J. Brouwer, M.A. van Gerven, F. P. de Lange, Prior expectations bias sensory representations in visual cortex. J Neurosci, 2013. 33(41): p. 16275-84.
​
21. J.W. Gibbs, Elementary Principles in Statistical Mechanics, developed with especial reference to the rational foundation of thermodynamics. New York: Charles Scribner & Sons, 1902.
​
22. C.E. Shannon, A Mathematical Theory of Communication. Bell Systems Technical Journal, 1948. 27(3): p. 379-423.
​
23. K. Friston, J. Kilner, L. Harrison, A free energy principle for the brain. J Physiol Paris, 2006. 100(1-3): p. 70-87.
​
24. F. Osinga, Science, Strategy, and War: The Strategic Theory of John Boyd. Eburon Academic Publishers, 2005.
​
​
​